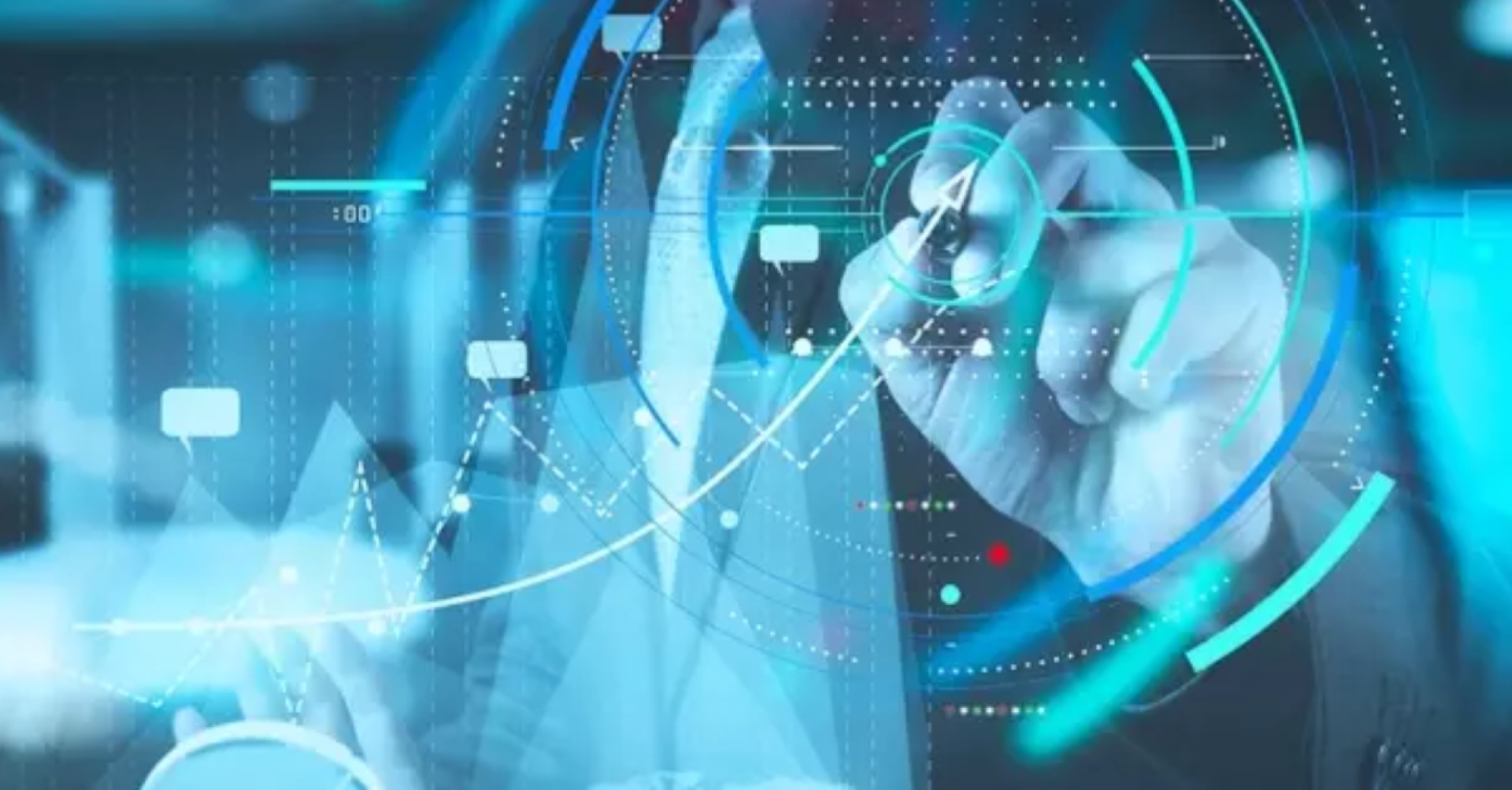
2nd February 2023
As a CEO of a data business in the UK, you understand the importance of staying ahead of the curve when it comes to delivering value to your customers. With the ever-increasing competition in the market, it's essential to continuously innovate and offer unique solutions that set your business apart. One way to do this is by harnessing the power of Machine Learning (ML) and Predictive Analytics.
ML and Predictive Analytics are rapidly becoming the backbone of data-driven businesses. They allow you to analyse vast amounts of data and uncover hidden patterns and trends that can help you better understand your customers and their needs (or ‘unmet needs’). This information can then be used to evolve your customer value proposition, delivering personalised and targeted solutions that meet their specific requirements.
In this article, we'll share insights from a CEO Forum we recently hosted, led by Deborah Kobewka, former CEO of Evaluate Pharma and a highly experienced operator within data-led businesses, on how data providers can transform their business models through the use of ML and Predictive Analytics.
1. Identifying the ‘Unmet Need’ of Customers
To develop predictive analytics products, data providers must first understand the unmet needs of their customers. For example, Evaluate Pharma consistently heard that pharmaceutical companies were looking for a better way to select the right molecules in the early stages of R&D, as the process can be long and expensive (approx $1bn), with a high risk of failure (90%). Evaluate Pharma set out to develop a product that would not only help customers understand the R&D risk profile of a new drug but also predict its commercial performance if passed through R&D and brought to market. Previous to this, on-demand access to predictive insights to assess drugs at the early stage of development would be to mandate a custom report to be written for c. $1m.
2. Adopting Machine Learning and Predictive Insights
To address an unmet need, a company must have a clear understanding of its data history and collect a wide variety of data attributes. In Evaluate Pharma's case, they set out to develop a product that helped customers understand the R&D risk profile of a new drug and predict how it might perform commercially if brought to market. Their data scientists were able to examine 20+ years of pharma asset data, with 50 attributes attached to each asset, and use machine learning to determine the most statistically significant indicators of commercial success. This became the basis for Evaluate's Omnium forecasting product.
3. Accessing Qualified Machine Learning Talent
One of the biggest challenges in adopting machine learning is finding the right talent. Most companies turn to third-party service providers for machine learning expertise during the initial exploration phase and then bring the function in-house once the concept is validated. Hiring a Head of Data Science should be the first step, as they can play both a team leader and senior technical expert role. The Head of Data Science will then build the team based on the state of the existing data. For example, if the data is not clean, they will first hire Data Engineers to improve its quality before hiring additional Data Scientists.
4. The Role of Leadership
It's crucial for the entire company to believe in the unmet need and focus on it. Business leaders must communicate the story consistently and pivot the company's strategy to support the unmet need.
5. Evolving Pricing to Reflect Increased Value
Pricing plays a crucial role in developing a new proposition. Customers will be willing to pay more if a business continues to add valuable data and analytics to its platform. There are two types of data propositions: those that level the playing field, and those that offer unique and differentiated value and offer customers a competitive advantage through the effective deployment of machine learning. It's important for data businesses to price their offerings appropriately to reflect the increased value they bring to customers.
6. Taking predictive products to market
Taking predictive products to market can be a challenge due to the complexity of the offering compared to just selling access to a database. The sales team will need to be educated and confident to effectively sell the product. One of the biggest questions customers will ask is for access to the algorithms. The answer must always be a resounding ‘no’, protecting algorithms is paramount to retaining IP and competitive advantage.
In conclusion, evolving your customer value proposition with ML and Predictive Analytics is a crucial step in the growth of your data business. By leveraging these technologies, you can deliver innovative and personalised solutions that meet the needs of your customers and set your business apart in the UK SME market
Keep innovating!